Introduction:
Taking into account how computerized reasoning examination implies to reproduce the working of the human mind – or what we are aware of it – in machines, it is nothing unexpected that AI scientists take motivation from the structure of the human cerebrum while making AI models. This is exemplified by the creation and utilization of counterfeit neural systems that are structured trying to reproduce the neural systems in our mind.
Similar to the multifaceted system of neurons that make up our cerebrum, a variety of similar neural units make up a profound neural system. These counterfeit neural systems, to a limited degree, have empowered machines to imitate the intellectual and coherent elements of the human mind. AI App Development Company would now consider the use of AI and implement it properly as and when needed.
There is a possibility that we see an ascend to AI frameworks that beat human specialists in picture acknowledgment and other comparative undertakings requiring examination and example acknowledgment required to recognize the substance of a particular picture, assemble content, or a sound clasp. Neural Network Development Company would now focus on implementing these in anything they design.
Just like our mind has various parts that empower various capacities, various types of neural systems are being created to take care of various types of issues. While there are various sorts of artificial neural systems being created and utilized by specialists, a couple have discovered more noteworthy appropriateness when contrasted with the rest.
In any case, before finding out about their various sorts, it is imperative to comprehend what neural systems are, the manner by which they work, and why they are significant. It is necessary to comprehend the fundamentals of neural systems and the most usually utilized models or kinds of artificial neural systems today.
Understanding artificial neural systems
Artificial neural systems structure the center of profound learning applications, a large portion of which are made to imitate the human personality’s capacity to recognize designs and decipher perceptual data. Neural systems are game plans of different hubs or neurons, orchestrated in numerous layers. The data enters the neural system through the info layer, which is the essential furthest layer.
The last layer through which data passes is the output layer. The information and output layers could possibly have extra layers between them. The layers, assuming any, present between the information and output layers are called concealed layers. A counterfeit neural system is viewed as a “profound” neural system on the off chance that it has different shrouded layers. By and large, every neuron in any layer is interconnected to all neurons in its nearby layers.
Each layer of the neural system separates the contribution to a less complex structure to decipher and order the substance. For example, consider a basic neural system that is utilized to distinguish the photos of felines. The various layers of the neural system perform various capacities and break down various components of information pictures. For instance, the primary later could just sweep for shapes in the pictures. The following layer can distinguish various hues.
So also, the ensuing layers can make progressively point by point investigations to distinguish increasingly unpretentious highlights, at last permitting the neural system to recognize the pictures of felines unmistakably. A high number of layers implies that there can be a higher number of pathways for data to go through the system, possibly permitting the system to perform profoundly complex assignments, for example, fast picture and video examination.
Neural systems gain the capacity to order pictures and articles in pictures through a procedure called preparing. Preparing neural systems allude to the way toward bolstering them with sets of information pictures and output names. For example, a neural system that can utilize CT filter pictures to analyze malignant growth, must be prepared by giving it huge volumes of CT examine pictures and revealing to it which pictures demonstrate the nearness of carcinogenic tumors and which ones don’t.
At the point when the neural system is prepared with enough combines of pictures and results, it recognizes the examples that are convincingly demonstrative of disease. The neural system at that point utilizes this preparation memory to analyze malignant growth utilizing CT checks.
1.Feedforward neural systems
Feedforward neural systems are the principal sort of counterfeit neural systems to have been made and can be considered as the most normally utilized ones today. These neural systems are called feedforward neural systems in light of the fact that the progression of data through the system is unidirectional without experiencing circles.
The quantity of layers relies upon the multifaceted nature of the capacity that should be performed. The single-layered feedforward neural system comprises of just two layers of neurons and no shrouded layers in the middle of them. Multi-layered perceptrons comprise of various concealed layers between the info and output layers, taking into account different phases of data preparing.
Feedforward neural systems discover applications in zones that require administered adapting, for example, PC vision. Feedforward neural systems are most normally utilized in object acknowledgment and discourse acknowledgment frameworks.
2. Recurrent neural network
Repetitive neural systems (RNN), as the name recommends, includes the repeat of tasks as circles. These are considerably more confounded than feedforward organizes and can perform more mind boggling assignments than essential picture acknowledgment. For example, repetitive neural systems are normally utilized in content forecast and language age. Understanding and producing common language includes substantially more intricate handling than picture acknowledgment, which intermittent neural systems can perform because of their engineering.
While in feedforward neural systems, associations just lead from one neuron to neurons in consequent layers with no criticism, repetitive neural systems take into account associations with lead back to neurons in a similar layer taking into consideration a more extensive scope of tasks.
In any case, customary RNNs have a couple of constraints. They are hard to prepare and have an extremely momentary memory, which confines their usefulness. To beat the memory constraint, a more current type of RNN, known as LSTM or Long Short-term Memory systems are utilized. LSTMs stretch out the memory RNNs to empower them to perform undertakings including longer-term memory.
3. Convolutional neural network
Convolutional neural systems, since the time its origination has only be related with PC vision applications. That is on the grounds that their design is explicitly appropriate for performing complex visual investigations. The convolutional neural system engineering is characterized by a three-dimensional game plan of neurons, rather than the standard two-dimensional cluster. The principal layer in such neural systems is known as a convolutional layer.
Every neuron in the convolutional layer just procedures the data from a little piece of the visual field. The convolutional layers are trailed by corrected layer units or ReLU, which empowers the CNN to deal with confounded data.
CNNs are primarily utilized in object acknowledgment applications like machine vision and in self-driving vehicles.
While these sorts of artificial neural systems are the most widely recognized in the present AI applications, there are numerous others that are being developed to accomplish a degree of usefulness that is increasingly similar to the human cerebrum.
Conclusion
Each new disclosure about our mind’s working prompts another achievement in the field of AI, prompting better models of neural systems. Subsequently, as we keep on understanding our cerebrums better, it is just a short time before we can repeat the totality of our mind’s working in PCs.
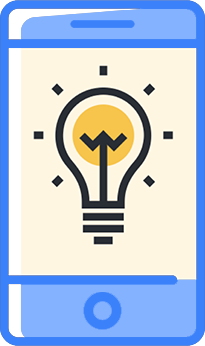